Neural network-based visual data compression
Abstract : Due to the continuous advances in display and acquisition technologies, huge amounts of diverse visual data are generated every day, which constitute a major issue in terms of storage and transmission. For this reason, visual data compression plays a prominent role in practice. A number of studies have been dedicated to the design of data compression methods, like the predictive and transform coding approaches adopted in different standards such as JPEG, JPEG2000, and HEVC. To further improve these conventional coding schemes, it becomes mandatory to design new coding tools. Recently, the use of neural networks and deep learning techniques have been well investigated for computer vision applications such as classification and recognition. In contrast, very little research has been done in the context of visual data compression. This special session aims to address the recent progress in neural network-based compression. More precisely, and in addition to standard image and video datasets, other kinds of visual data, like stereo/multi-view images and light fields, can be considered. Moreover, unlike recent works focusing on intra and/or inter prediction steps and the associated entropy coding module arising in a typical compression scheme, one novelty in this special session will consist of the investigation of transform learning approaches for coding purposes. Thus, this session will be an opportunity for researchers and engineers working on this active field to share and discuss their recent works. Topics of interest in this special session include various challenges related to neural network-based visual data compression such as learning wavelet filters, designing advanced nonlinear transform coding techniques, proposing new methods for intra/inter prediction, rate allocation, and entropy coding.
Organizers
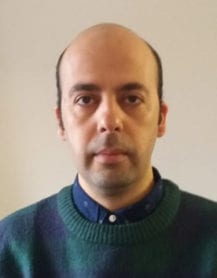
Mounir Kaaniche
Univ. Paris 13
France
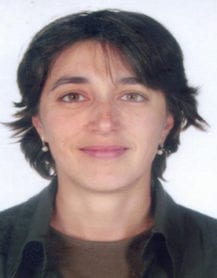
Amel Benazza-Benyahia
SUP’COM-Tunis
Tunisia