Plenary Speakers
Prof. Lina Karam 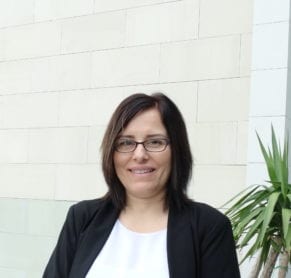
Dean of the School of Engineering, Lebanese American University
Towards Resilient Deep Learning
While deep neural networks (DNNs) perform on par with – or better than – humans on pristine high-resolution images, DNNs were shown to be very sensitive to small adversarial perturbations even when the perceived visual quality of the images is not affected. Furthermore, DNNs were found to be sensitive to images with quality degradations, which are frequently encountered in real-world applications. While recent advances in machine learning may help increase the resilience of DNNs, many challenges remain to be addressed. This plenary presents a selective review of visual quality factors and adversarial attacks that were found to significantly affect the DNN recognition performance. This plenary will also cover promising “defense” methods and will offer insights into remaining challenges.
Prof. Lina J. Karam is the Dean of the School of Engineering and Professor of Electrical and Computer Engineering at the Lebanese American University. She is an IEEE Fellow and Editor-In-Chief of the IEEE Journal on Selected Topics in Signal Processing (IEEE JSTSP). Prior to joining LAU, Prof. Karam was a Full Professor in the School of Electrical, Computer & Energy Engineering, Arizona State University, where she also served as Computer Engineering Director for Industry Engagement. Prof. Karam was awarded a U.S. National Science Foundation CAREER Award, a NASA Technical Innovation Award, IEEE Region 6 Award, IEEE SPS Best Paper Award, Intel Outstanding Researcher Award, and IEEE Phoenix Section Outstanding Faculty Award. Prof. Karam’s industrial experience includes video compression R&D at AT&T Bell Labs, multidimensional data processing and visualization at Schlumberger, and collaborations on computer vision, machine learning, image/video processing, compression, and transmission projects with various industries. She served as General Chair of IEEE ICIP 2016 and as General Co-Chair of IEEE ICME 2019. She helped initiate the World’s First Visual Innovation Award that was presented for the first time at IEEE ICIP 2016 and more recently the World’s First Multimedia Star Innovator Award that was presented at IEEE ICME 2019. Prof. Karam has over 220 technical publications and she is an inventor on 7 issued US patents. She served on the IEEE Publication Services and Products Board (PSPB) Strategic Planning Committee, IEEE SPS Board of Governors, IEEE CAS Fellow Evaluation Committee, and the IEEE SPS Conference Board. In addition to serving as EiC of IEEE JSTSP, Prof. Karam is currently serving on the IEEE TechRxiv Advisory Board, IEEE Access Journal Editorial Board, IEEE SPS Awards and Publications Boards, IEEE SPS IVMSP TC, and IEEE CAS DSP TC.
Prof. Katherine L. (Katie) Bouman 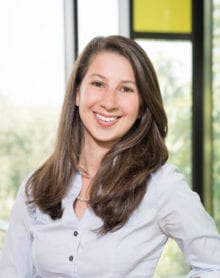
Assistant Professor, Department of Computing and Mathematical Sciences, California Institute of Technology
Capturing the First Picture of a Black Hole & Beyond
This talk will present the methods and procedures used to produce the first image of a black hole from the Event Horizon Telescope, as well as future developments. It had been theorized for decades that a black hole would leave a “shadow” on a background of hot gas. Taking a picture of this black hole shadow would help to address a number of important scientific questions, both on the nature of black holes and the validity of general relativity. Unfortunately, due to its small size, traditional imaging approaches require an Earth-sized radio telescope. In this talk, I discuss techniques the Event Horizon Telescope Collaboration has developed to photograph a black hole using the Event Horizon Telescope, a network of telescopes scattered across the globe. Imaging a black hole’s structure with this computational telescope required us to reconstruct images from sparse measurements, heavily corrupted by atmospheric error. This talk will summarize how the data from the 2017 observations were calibrated and imaged, and explain some of the challenges that arise with a heterogeneous telescope array like the EHT. The talk will also discuss future developments, including how we are developing machine learning methods to help design future telescope arrays.
Prof. Katherine L. (Katie) Bouman is a Rosenberg Scholar and an assistant professor in the Computing and Mathematical Sciences and Electrical Engineering Department at the California Institute of Technology. Her research focuses on computational imaging: designing systems that tightly integrate algorithm and sensor design, making it possible to observe phenomena previously difficult or impossible to measure with traditional approaches. This work led her to be a key member of the international Event Horizon Telescope Collaboration, which in April 2019 imaged the first-ever a picture of the Messier 87 (M87) black hole fifty-five million light-years away. Prof. Bouman’s group at Caltech combines ideas from signal processing, computer vision, machine learning, and physics to find and exploit hidden signals for both scientific discovery and technological innovation. Prof. Bouman received her undergraduate degree from the University of Michigan, and completed her Master’s and PhD at the Massachusetts Institute of Technology (MIT), in Cambridge, Massachusetts. She is formerly a Postdoctoral Fellow at the Center for Astrophysics | Harvard & Smithsonian. Recently she was a recipient of the Breakthrough Prize in fundamental physics as part of the Event Horizon Telescope team.
Prof. Wolfgang Heidrich 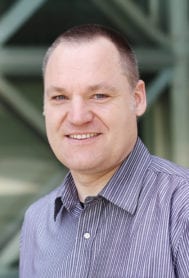
Director of the Visual Computing Center, King Abdullah University of Science and Technology.
Learned Optics — Improving Computational Imaging Systems through Deep Learning and Optimization
Computational imaging systems are based on the joint design of optics and associated image reconstruction algorithms. Historically, many such systems have employed simple transform-based reconstruction methods. Modern optimization methods and priors can drastically improve the reconstruction quality in computational imaging systems. Furthermore, learning-based methods can be used to design the optics along with the reconstruction method, yielding truly end-to-end optimized imaging systems that outperform classical solutions.
Prof. Wolfgang Heidrich is a Professor of Computer Science and the Director of the Visual Computing Center at King Abdullah University of Science and Technology (KAUST), as well as the interim leader for KAUST’s AI Initiative. Prof. Heidrich joined KAUST in 2014, after 13 years as a faculty member at the University of British Columbia. He received his PhD in from the University of Erlangen in 1999, and then worked as a Research Associate in the Computer Graphics Group of the Max-Planck-Institute for Computer Science in Saarbrucken, Germany, before joining UBC in 2000. Prof. Heidrich’s research interests lie at the intersection of imaging, optics, computer vision, computer graphics, and inverse problems. His more recent interest is in computational imaging, focusing on hardware-software co-design of the next generation of imaging systems, with applications such as High-Dynamic Range imaging, compact computational cameras, hyperspectral cameras, to name just a few. Prof. Heidrich’s work on High Dynamic Range Displays served as the basis for the technology behind Brightside Technologies, which was acquired by Dolby in 2007. Prof. Heidrich has served on numerous program committees for top-tier conferences such as Siggraph, Siggraph Asia, Eurographics, EGSR, and he has chaired the papers program for both Siggraph Asia and the International Conference of Computational Photography (ICCP) among others. Prof. Heidrich is the recipient of a 2014 Humboldt Research Award.