Learning-based Processing of 3D Visual Data
Abstract : 3D visual data, including point clouds, lightfield images, 360 images and video, are emerging as pivotal data type in multiple fields, such as robotics, computer graphics, medical industry and many more. This kind of data is gaining attention due to increased availability of instruments such as LiDARs and lightfield cameras and disruptive applications such as autonomous driving. There is great interest in exploiting the richness of the 3D geometrical representation, but 3D visual data processing can be very complex and capturing all the nuances with a model-based approach can be very challenging. For this reason, the field calls for novel learning-based techniques. Learning-based approaches to processing 3D data are still few and less refined with respect to mature fields such as traditional image processing. The goal of this special session is to bring together experts from academia and industry to share novel ideas and approaches for this challenging task. Topics of interest for this session include but are not limited to:
– Neural networks for point cloud classification, segmentation, enhancement and compression
– Graph learning methods for 3D inverse problem regularization
– Metric learning for quality assessment of 360-degree images and video
– Lightfield compression and enhancement
Organizers
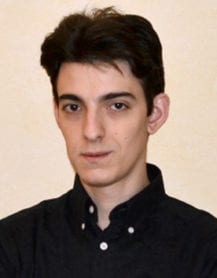
Diego Valsesia
Politecnico di Torino
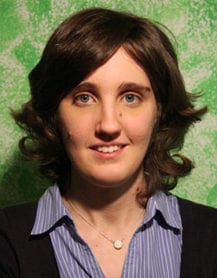
Giulia Fracastoro
Politecnico di Torino
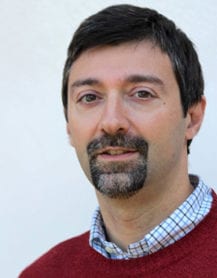
Enrico Magli
Politecnico di Torino