Explainable Machine Learning for Image Processing
Abstract : Explainability of deep neural networks (DNNs) refers to the revelation of what has compelled the model to make a specific decision. Explainability not only helps with improving the model by knowing what exactly is going on in the network, but also facilitates detecting the failure points of the model. No matter how powerful DNNs are, they will not be used in practice unless they can be interpreted and related to the image landmarks used by humans. Explainable Artificial Intelligence (XAI) becomes even more important for image processing in specific application domains such as medical imaging, as not a single mistake is allowed in medical decisions. Potential mistakes may lead to irreparable loss or injury, and knowing the logic behind the outcome of the model is the key for image-based prognostics and diagnostics. Although there have been significant advancements in improving the interpretability of DNNs, it is still only heuristically understood, and further reliable explanations need to be developed. The objective of this special session is to collect novel ideas and experiments on how to enhance explainability of DNNs and solve the black box problem, which is a barrier to make use of these models in real world applications.
Organizers
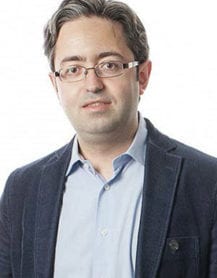
Arash Mohammadi
Concordia University
Canada
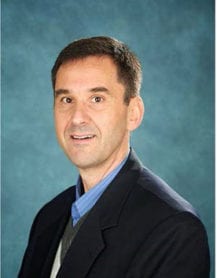
Konstantinos N Plataniotis
University of Toronto
Canada
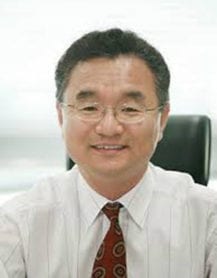
Yong Man Ro
KAIST
South Korea