Dynamic Background Reconstruction/Subtraction for Challenging Environments
Abstract: Segmentation of moving foreground objects is crucial for surveillance of human activities, visual observation of animals, and human-machine interaction. Since the beginning, different well-known background modeling approaches made their own breakthrough such as Gaussians models, sample-based methods and robust subspace learning models. Many scientific efforts have been reported in the literature to improve leader or classical methods in a more progressive way in applications where challenges are becoming more complex. However, the top background subtraction methods currently compared in CDnet 2014 are based on deep neural networks, and have demonstrated a large performance improvement in comparison to conventional unsupervised approaches. But, their main drawbacks are their computational and memory requirements, and also their supervised aspects requiring labeling of a large amount of data. The goals of this special session are thus three-fold: 1) designing background subtraction algorithms for real applications in urban and natural environments; 2) proposing new adaptive and incremental algorithms using supervised or unsupervised machine learning models; and 3) proposing robust algorithms to handle the key challenges in background subtraction in the case of either static or moving cameras. Papers are solicited to address background modeling and subtraction algorithms based on mathematics, machine learning and signal processing theories.
Organizers
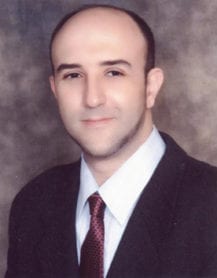
Naoufel Werghi
Khalifa University
United Arab Emirates.
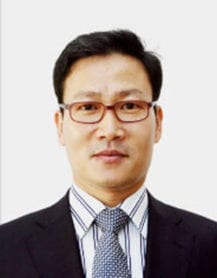
Soon Ki JungKyungpook
National University (KNU)
Korea.
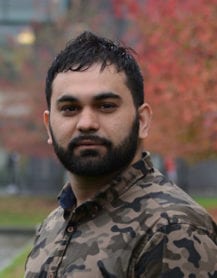
Sajid Javed
Khalifa University
United Arab Emirates.
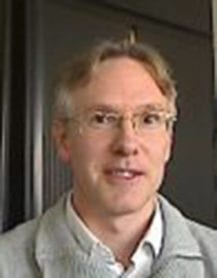
Thierry Bouwmans
La Rochelle Université
La Rochelle, France.
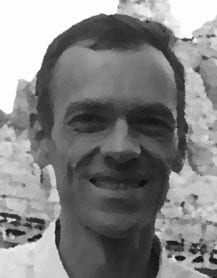
Marc Van Droogenbroeck
Université de Liège
Belgium.