Advanced Image/Video Coding for Machine Vision
Abstract : Image/video data occupies more than 80% of all Internet traffic, driving research to further improve compression efficiency to reduce the storage and transmission costs. Traditional coding approaches target the best image/video quality under certain bit-rate constraints and are optimized for viewing by humans. However, a greater amount of image/video data is being captured and transmitted for analysis by machines, and there is not necessarily a need to view the resulting images/videos with high fidelity. This new paradigm is being driven by emergence of connected vehicles and IoT devices, very large-scale video surveillance networks, smart cities, and quality inspection, all of which have stringent requirements on latency and scale, demanding new solutions for image/video coding that target machine vision.
The requirements for machine vision have inspired new research directions and approaches that represent a significant departure from existing visual coding research. For instance, recent advances in deep learning for various classification and inference tasks have motivated studies on the relation between suitable compressed representations and effectiveness of machine vision algorithms, as well as further study on compact features. These new representations are expected to greatly reduce the transmission cost compared to state-of-the-art compression schemes, while providing the necessary information for machine vision system to operate with high accuracy, at large scales and in a distributed manner.
This special session seeks submissions about the latest advances in image/video coding for machine vision. We especially encourage novel image/video coding approaches for machine vision, including deep learning-based techniques and compact feature representations. We also welcome contributions related to the robustness of particular representations to adversarial attacks as well as privacy-preserving representations for machine vision. Topics of interest of this special session include, but are not limited to:
- Learned Image/Video Compression for Machine Vision
- Feature Compression Technology
- Compact Descriptors for Visual Analytics
- Semantic-Oriented Compression
- Coded Vision Technology
- Collaborative or Adversarial Learning for Machine Vision
- Privacy-Preserving Representations for Machine Vision
- Scalable and Distributed Architectures for Machine Vision
Organizers

Jiaying Liu
Peking University
China
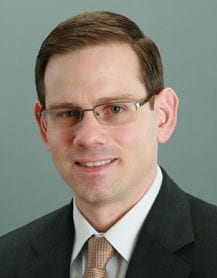
Anthony Vetro
MERL
USA
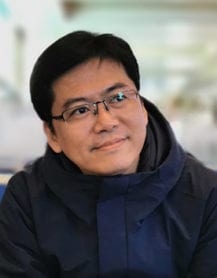
Ling-Yu Duan
Peking University
China
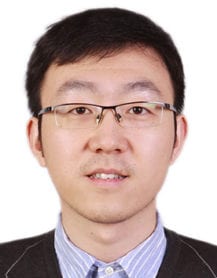
Dong Liu
Uni. of Sci & Tech of China
China